Can a shock rock?
Events are changing the way we think about data and technology
This is the fourth and final article in our adaptive model series.
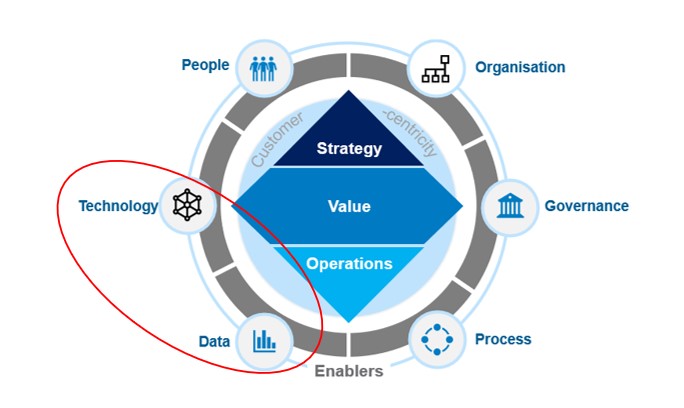
The “oil shock” of the early 1970s rocked the foundations of the automotive industry, especially manufacturers of large cars with thirsty engines.
Fuel shortages and skyrocketing prices caused people to rethink radically – and recalibrate the type of car they needed. Suddenly, small was big.
In Australia, the oil shock led to the demise of Leyland and its new flagship sedan, the P76, which, with unintended irony, boasted that a 44-gallon drum would fit in its boot (trunk). The Leyland company was sunk in Australia less than 18 months after the launch of the P76.
Similar changes took place around the world. But some manufacturers were much better placed to respond to the emerging conditions. The Japanese had already focused on small, efficient, reliable cars. Indeed, reliability was part of the competitive frontier at the time. And the oil shock catapulted the humble VW Beetle and its stablemate, the Golf (Rabbit in the US), to the sales stratosphere. The Love Bug and other Herbie movies no doubt helped too.
Much more recently we have also been experiencing a series of shocks, and the story of the automotive industry is instructive when thinking about technology and data.
The shocks keep coming
The last three years have seen levels of disruptive change that are historically unprecedented. Starting with the pandemic, continuing with the impacts of wars, then inflation and latterly, the arrival of generative AI in forms that can revolutionise many approaches to doing business. These shocks may turn out to be good for us.
Our big technology transformation projects are like the V8 muscle cars of the 1970s – lots of power, which can be exhilarating, so long as you go in a straight line and don’t need to change course in a hurry.
Big transformation projects take a long time – often years – are resource intensive, and do not handle “bumps in the road” effectively. Moreover, the expectation is that the entire business will reinvent itself to fit with the new technology platform. So things can become problematic, and that is before we consider the spectacular failure rate of big technology projects.
Indeed, the very best ‘target operating model’ (TOM) programs do a pretty good job of defining the ‘to-be’ state and keeping on track to get there. However, it’s rarely the case that these programs recognise the need to alter direction rapidly, in response to changing external conditions.
Yes, there’s the agile approach, but that tends to focus on technology change rather than ‘model change’, and change programs that purport to be built on agile principles are not immune to failure.
It is also interesting to note that customer-facing technologies (think banking apps and online shopping) are afforded significant and often very successful investments in purpose-made, highly tailored platforms and solutions. By contrast, procurement and other back-office functions are typically expected to make do with off-the-shelf solutions.
We cannot afford to keep forcing corporate functions to re-engineer themselves fundamentally in order to accommodate generic, off-shelf technology solutions. Thankfully, we no longer need to.
Today, one of the most important factors we need to embrace is the speed at which technology is advancing. Everything is changing. And it is changing for the better.
The ability to develop bespoke technology at an enterprise level is now with us. With the advent of AI, much of the ‘tailoring task-load’ can be automated and accelerated. And it will reduce the time to achieve the desired outcomes. It should also, if managed intelligently, de-risk the process, as digital workers are less likely to make mistakes.
We should also keep in mind that the owners of the off-shelf generic solutions will need to respond to this new competition from bespoke solutions. They will need to offer a far more flexible tailoring service for their product. The smart generic providers are already looking at this, as well as investing in the very AI capabilities that will make a bespoke build more feasible. There will be a convergence. The adaptive model is ideally suited to the changes that have started and are yet to come.
We need to make data more meaningful
Traditional transformation approaches are often found wanting when it comes to embracing the power and potential of data to provide management information (MI). Many start their change journey data rich, but MI poor. Then they emerge from the transformation in exactly the same place after months, possibly years, of effort.
We know that good data is the bedrock of great information, insights and decisions. Yet most businesses still struggle with finding data and turning it into something useful. Far too many poor procurement decisions can be traced back to causes that include poor MI.
Even organisations that manage to develop meaningful MI often see it wasted, as it isn’t easy to find, and far too often it is not presented convincingly.
The key to unlocking these problems involves fixing both the back-end data and the front-end visualisation experience. The former generally resides within the organisation’s ERP platforms and the latter is increasingly becoming potentially fix-able via the self-serve capabilities of tools such as Business Objects (SAP), Power BI (Microsoft), Qlik Sense and other solutions. These tools generally permit significant customisation so that users can pull the information at the point of need, or have it pushed to them based on business rules.
Fixing the data-to-MI problem is one of the most common challenges faced by procurement organisations but there’s now no excuse for this to be a problem within an adaptive operating environment.
Organisations that recognise this are most likely to effect change successfully. They will be successful by embracing the adaptive potential of their technologies and the valuable MI now readily available to users, as and when they need it.
And, finally, circling back to the earlier point about automation, it’s most certainly the case that the human-oriented “grunt” will be progressively removed from the processes of organising, extracting, preparing, and analysing data, in the coming months and years. These processes are ripe for both machine learning and automation. So, such tasks will be quick, cheap and error-free. Above all, the change should be adaptive.