BUSINESS TRANSFORMATION
The role of data annotation in the development of AR applications
Augmented reality (AR) applications combine the physical and digital worlds by adding digital objects to the real world. AI technologies for fields such as computer vision, object recognition, speech recognition, NLP, and translation have AR applications that enhance the applications and provide a more compelling user experience. AI is used for object recognition, facial recognition, text recognition and translation, and other features in AR.
The foundation these Artificial Intelligence (AI) technologies operate on is the colossal volume of data used to train them. Consequently, the efficiency and accuracy of AR applications depend on how well the data has been annotated to fit the requirements of the AI technology thus, enabling them to make accurate predictions and classifications.
What is annotation and labelling?
Data annotation is the technique through which the data is labelled to make objects recognisable by machines. Data labelling is all about adding more metadata to various data types (text, audio, image, and video) to train Machine Learning (ML) models. It is a part of data science and plays a critical role in the development and accuracy of AI and ML models.
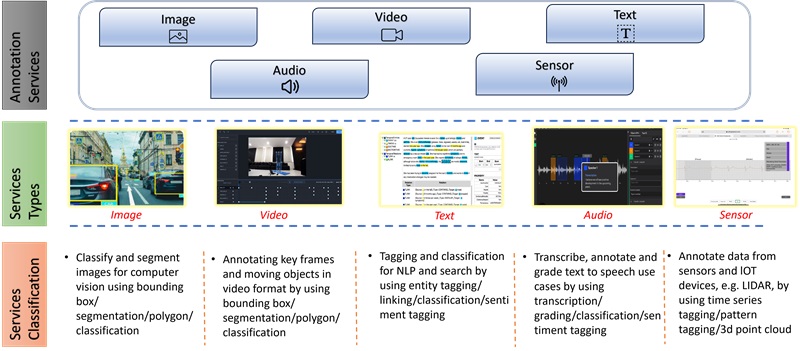
So, how is data annotated for AR applications?
Image Annotation:
Object recognition and facial recognition involve data image annotation. Image data annotation is carried out using methodologies such as bounding boxes, lines, polygonal segmentation, splines, semantic segmentation, 3D cuboid, and landmark.
Let us take a quick look at a few of these methods
The bounding box method labels objects in an image within a rectangle. Retail AR apps use it to identify products and guide customers to them. Objects which do not fit into a rectangular shape are labelled using polygonal segmentation. For example, we may have several images of street scenes in which we want to identify trucks, pedestrians, bicycles, or cars. It is possible to annotate them separately on the same image either by using a bounding box or polygon.
Semantic segmentation is a more advanced application of image annotation. It is used to analyse the visual content of images and determine how objects in an image are different or the same. This method is used when we want to understand the presence, location and sometimes the size and/or shape of objects in images. For example, we have several images including a crowd and a stadium which we want to annotate, it is possible to annotate the crowd to segment the stadium seats.
Deep learning image segmentation makes it possible to track and count the presence, position, number, size, and shape of objects in an image. Using the previous example of the stadium and the crowd, it is possible to annotate both the individuals in the stadium and to determine the number of people in the crowd using this type of annotation and per-pixel segmentation. It is also one of the data annotation methods for facial recognition as it provides information of fine granularity.
Phone apps with face animation use facial recognition. Landmark annotates key points or dots within the image. Counting applications use it to determine the density of the target object in an image, for example, locating and counting the number of zombies in an AR game. It is also used for gesture and facial identification.
3D cuboid involves annotating objects in 2D images to get a three-dimensional aspect using height, width, and depth. A furniture retailer's space planning app can use the 3D cuboid method to display how furniture products fit into a customer's home. Line and spline annotation labels the image with either straight lines or curves. Gaming AR apps can use line and spine to demarcate pavements, road lanes, and other road marks.
Video Annotation
Unlike image annotation, video annotation involves annotating objects on a frame-by-frame basis to make them recognisable for ML models. It is a process of adding feedback or extra information to a respective video. This can be in the form of text, shapes, or timestamps and serves various purposes, such as enhancing viewer engagement or clarifying content. An AR app that functions as a guide is a use case for video annotation. These apps overlay complicated layouts with information allowing users to quickly reach their destination in hotels, museums, or malls.
Audio Annotation
Audio annotation is a subset or sub-division of data annotation that involves the classification of components of audio that originate from people, animals, instruments, etc. Audio data annotation can be of several types - speech-to-text transcription, sound labelling, event tracking, audio classification, natural language processing (NLP), and music classification. NLP annotates human speech focusing on nuances like dialect, semantics, tone, and context. The best-known examples of NLP, which have increasingly integrated into our lives are Siri, Alexa, etc. AR apps can use speech-to-text transcription to provide a feature that converts users' verbal comments as displayed overlaid text in a remote assistance AR app or a tourist venue AR app.
Text Annotation
Text annotation consists of sentiment, intent, semantic, entity, and linguistic labelling. Sentiment labelling classifies whether the data has a positive, negative, or neutral overtone. Intent labelling categorises the aim behind the text as a command, request, or confirmation. Semantic labelling adds metadata to the subject discussed to understand the concept. Entity labelling marks parts of speech, named entities, and key phrases in the text. Linguistic labelling pinpoints grammatical elements to understand the context and the subject being discussed across multiple sentences. AR tutor apps use text annotation to provide further explanations for a passage that the student has highlighted. Gaming and AR maps use text labelling to read street and traffic signs. Text annotation is used in combination with audio annotation in AR for education, remote assistance, and manufacturing.
AR is now maturing into a utility and is no longer a niche solution. Varied use cases across industries are being conceived with the advancements in technology. Data annotation is a cornerstone in the growth of AR, as the solution's core functionality depends on the training data's quality. The focus on and demand for data annotation will continue as the process of adding extra virtual information to an object, is one of the most common uses for AR thus, allowing the AR applications to understand and network with the real world in a better and more expressive way.