BUSINESS TRANSFORMATION
The Real Challenges of Data Accessibility in Outsourcing
Data is currently at the center of everything we do. No business can thrive without it. Outcomes depend on the quantity and quality of data you can access and leverage to improve timelines and operations.
Data seems to be available at a large scale and in various forms, but accessing it comes with many challenges to businesses, especially when the book of work is outsourced. To harness the true value of this data, businesses need a way to access it.
Data accessibility refers to how an individual (employee, vendor or organization) can leverage available data to improve production efficiency and provide business value/insights. Outsourced data analytics is majorly impacted due to data accessibility challenges.
Data and outsourcing: an accessibility challenge
Outsourcing data for transformation poses several challenges for organizations. Ensuring data privacy, maintaining regulatory compliance and managing third-party risks are key concerns. Some major challenges are:
- Third-party providers may face security breaches, making the confidentiality and integrity of outsourced data questionable.
- Meeting data protection regulations and industry standards across different jurisdictions can be complex, leading to compliance challenges. For instance, the General Data Protection Regulation (GDPR) provides individuals within the EU with greater control over their personal data and imposes obligations on organizations regarding its collection, storage and processing. Health Insurance Portability & Accountability (HIPAA) regulates the use of personal health information (PHI) by healthcare providers and ensures confidentiality. The Personal Data Protection Bill (PDPB) regulates the processing of personal data of individuals in India.
- Determining the data owner and maintaining control over the usage of data becomes critical, raising issues around governance and accountability.
- Transferring data seamlessly and integrating it into existing systems can be problematic, impacting accessibility and usability.
- Dependence on external vendors introduces the risk of service interruption, data loss or other issues that may affect data accessibility.
- Ensuring that data can be easily moved or accessed when needed, especially during a transition between outsourcing partners.
- Consistency in data quality across different outsourced processes and systems.
Addressing these challenges requires careful planning, robust contracts and continuous monitoring to ensure data accessibility while mitigating risks.
Mitigating data accessibility risks
Here’s what you can do to minimize the risks of data accessibility:
- Draft comprehensive contracts and Service Level Agreements (SLAs) defining data access requirements, security measures and penalties for non-compliance.
- Implement robust encryption protocols to protect sensitive data during transfer and storage, reducing the risk of authorized access.
- Classify data based on sensitivity, allowing for tailored security measures and ensuring that critical information receives enhanced protection.
- Stay informed about data protection laws in relevant markets or regions, and ensure that outsourcing practices align with legal requirements.
- Clearly define data ownership in contracts, specifying the rights and responsibilities of both the outsourcing entity and the service provider.
- Implement a robust vendor risk management program, assessing the reliability and security measures.
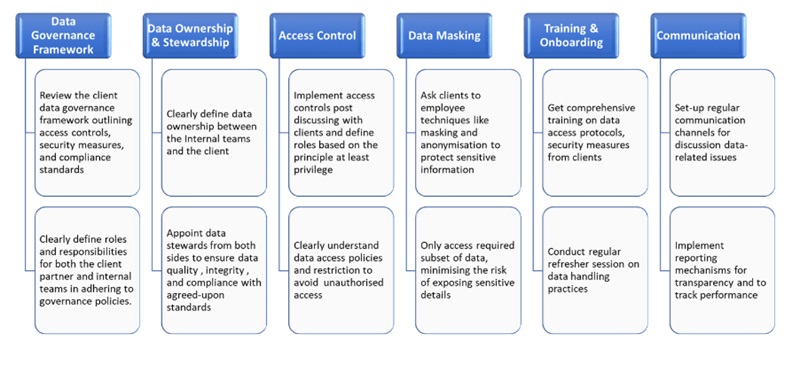
To address data accessibility challenges in outsourcing, consider dividing roles and activities in a structured manner. This can help client organizations and outsourcing partners minimize data accessibility challenges in outsourcing while maintaining data integrity and security.
Synthetic data using Gen AI: a new approach towards data accessibility
Creating synthetic data using generative AI involves leveraging algorithms and models to generate data that resembles real-world datasets but doesn’t contain actual sensitive information.
Though useful, synthetic data comes with hurdles of its own:
- Ensuring synthetic data accurately represents the complexity and nuances of real-world scenarios.
- Creating synthetic data that generalizes itself to various situations and diverse conditions.
- Introducing biases in synthetic data that mimic biases present in the training data leads to skewed model outcomes.
- Verifying the quality and effectiveness of synthetic data in comparison to real data poses a challenge, requiring robust validation methods.
- Balancing the generation of useful data and privacy preservation, especially when dealing with sensitive information.
In order to navigate the aforementioned challenges, organizations should do the following:
- Understand the target dataset thoroughly to ensure synthetic data mirrors its characteristics.
- Generate diverse samples, including edge cases, to enhance model robustness and avoid overfitting to specific patterns.
- Use validation metrics to assess how well synthetic data replicates real-world scenarios, ensuring it aligns with the target distribution.
- Implement techniques such as differential privacy or generative models that safeguard sensitive information while creating realistic data.
- Be vigilant of biases in the synthetic dataset and take measures to correct them, ensuring fairness and preventing model biases.
- Collaborate with domain experts to refine synthetic data generation, incorporating their insights to enhance authenticity.
- Augment real datasets with synthetic samples to strike a balance between realism and diversity, improving overall model performance.
Simplified guide for synthetic data using Gen AI
The following are a set of tips that will help organizations navigate the complexities of synthetic data using generative AI.
- Identify the key features and patterns present in your real data that you want to replicate synthetically.
- Select a generative AI model suitable for your needs, like Generative Adversarial Networks (GANs), Variational Autoencoders (VAEs) or another deep learning model.
- The model learns the underlying patterns and structures in the data during the training process based on a real dataset. Once the model is trained, use it to generate synthetic data. The data should mimic the statistical properties and patterns of your original dataset.
- Assess the quality of the synthetic data by comparing statistical properties, distributions and patterns with the original data. Refine the model if needed.
- Ensure the synthetic data doesn’t contain any identifiable or sensitive information. It should comply with data privacy regulations and ethical standards.
- Employ the synthetic data for testing, analysis or development purposes where using real data may not be feasible.
The effectiveness of synthetic data depends on the complexity and uniqueness of the original dataset, so continuous refinement may be necessary.
Ensuring effective data accessibility involves navigating a complex landscape of challenges and solutions. From addressing security concerns in outsourcing, to implementing robust encryption techniques, organizations must carefully balance accessibility with privacy and compliance, while staying informed about emerging solutions.
Ultimately, a holistic approach that considers legal, ethical and technological aspects is crucial for fostering data accessibility while safeguarding sensitive information.